Retrieval PDF
PDF 문서 embedding - vectorize db와 비교 - similar score - retreival document
(1) Pagkage Import
from langchain.vectorstores import Chroma
from langchain.text_splitter import RecursiveCharacterTextSplitter
from langchain.llms import OpenAI
from langchain.chains import RetrievalQA
from langchain.document_loaders import TextLoader
from langchain.document_loaders import PyPDFLoader
from langchain.document_loaders import DirectoryLoader
# Embedding
# !pip install InstructorEmbedding
from InstructorEmbedding import INSTRUCTOR
from langchain.embeddings import HuggingFaceEmbeddings
# Call openAI API usage
from langchain.callbacks import get_openai_callback
(2) Load text files
- 하나의 텍스트만 부를 경우
TextLoader
사용
# pip install pypdf
loader = DirectoryLoader('./pdf',glob="./*.pdf",loader_cls=PyPDFLoader)
documents = loader.load()
len(documents) # 13
PDF :
https://arxiv.org/abs/2004.02334
Finding the Optimal Vocabulary Size for Neural Machine Translation
We cast neural machine translation (NMT) as a classification task in an autoregressive setting and analyze the limitations of both classification and autoregression components. Classifiers are known to perform better with balanced class distributions durin
arxiv.org
(3) text를 max token에 맞춰 분절
# splitting the text into
text_splitter = RecursiveCharacterTextSplitter(chunk_size=1000, chunk_overlap=200)
texts = text_splitter.split_documents(documents)
print(texts[2].page_content)
Since the parameters of modern machine learn-
ing (ML) classification models are estimated from
training data, whatever biases exist in the training
data will affect model performance. Among those
biases, class imbalance is a topic of our interest.
Class imbalance is said to exist when one or more
1Tools, configurations, system outputs, and analyses are at
https://github.com/thammegowda/005-nmt-imbalanceclasses are not of approximately equal frequency
in data. The effect of class imbalance has been
extensively studied in several domains where clas-
sifiers are used (see Section 6.3). With neural net-
works, the imbalanced learning problem is mostly
targeted to computer vision tasks; NLP tasks are
under-explored (Johnson and Khoshgoftaar, 2019).
Word types in natural language models resemble
a Zipfian distribution, i.e. in any natural language
corpus, we observe that a type’s rank is roughly
inversely proportional to its frequency. Thus, a
few types are extremely frequent, while most of
(4) HuggingFace Instructor Embedding
- Embedding
- OpenAIEmbedding 사용시 퀄리티는 보장하지만 비용이 증가한다. HuggingFace의 HuggingFaceInstructEmbeddings을 사용해 절약 할 수 있다.
from langchain.embeddings import HuggingFaceInstructEmbeddings
instructor_embeddings = HuggingFaceInstructEmbeddings(model_name="hkunlp/instructor-xl",model_kwargs={"device":"mps"}) # !=mac "device":"cuda:0"
# load INSTRUCTOR_Transformer
# max_seq_length 512
(5) Create DB
# Embed and store the texts
# Supplying a persist_directory will store the embeddings on disk
persist_directory = "pdf_db"
## here we are using OpenAI embeddings but in future we will swap out to local embeddings
embedding = instructor_embeddings
vectordb = Chroma.from_documents(documents=texts, embedding=embedding, persist_directory=persist_directory)
(6) persiste (사용 db 유지)
vectordb.persist()
vectordb = None
vectordb = Chroma(persist_directory=persist_directory, embedding_function=embedding)
(7) Make a retriever
retriever = vectordb.as_retriever()
(8) retriever relevant documents
- 참조할 문단 수(k=3)
docs = retriever.get_relevant_documents("What is BERT Optimal Vocabulary Size?")
len(docs) # 2
retriever = vectordb.as_retriever(search_kwargs={"k":3})
(9) Define Util
- 새로 추가된 Langchain.callback - get_openai_callback을 통해 사용량을 볼 수 있다.
import textwrap
def wrap_text_preserve_newlines(text, width=110):
lines = text.split("\n")
# wrap each line individually
wrapped_lines = [textwrap.fill(line, width=width) for line in lines]
# Join the wrapped lines back together using newlines characters
wrapped_text = '\n'.join(wrapped_lines)
return wrapped_text
def process_llm_response(text):
with get_openai_callback() as cb:
llm_response = qa_chain(text)
print(wrap_text_preserve_newlines(llm_response['result']))
print('\n\nSources:')
for source in llm_response['source_documents']:
print(source.metadata['source'])
print('\n')
print(cb)
(10) 어떤 Tokenizer를 사용했는지 질문 - (한글은 답변이 어렵다고 회피)
query = "which tokenizer use in paper?"
process_llm_response(query)
BPE (Byte Pair Encoding) vocabulary.
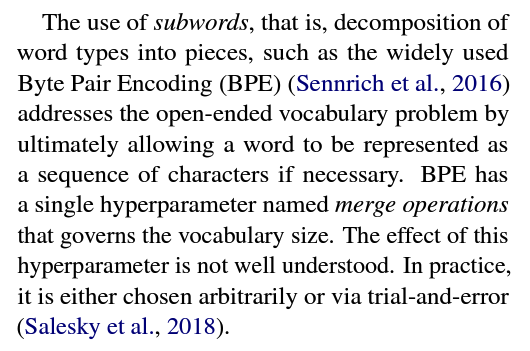
'🗣️ Natural Language Processing' 카테고리의 다른 글
[LangChain] No using OpenAI API RetrievalQA (0) | 2023.05.28 |
---|---|
[Mac] Transformer model downloaded path (0) | 2023.05.28 |
[LangChain] Building Custom Tool (0) | 2023.05.24 |
small scale text data classification (0) | 2023.05.16 |
[M1 Transformers] M1 Mac Transformers Install Error (0) | 2022.06.19 |
Retrieval PDF
PDF 문서 embedding - vectorize db와 비교 - similar score - retreival document
(1) Pagkage Import
from langchain.vectorstores import Chroma
from langchain.text_splitter import RecursiveCharacterTextSplitter
from langchain.llms import OpenAI
from langchain.chains import RetrievalQA
from langchain.document_loaders import TextLoader
from langchain.document_loaders import PyPDFLoader
from langchain.document_loaders import DirectoryLoader
# Embedding
# !pip install InstructorEmbedding
from InstructorEmbedding import INSTRUCTOR
from langchain.embeddings import HuggingFaceEmbeddings
# Call openAI API usage
from langchain.callbacks import get_openai_callback
(2) Load text files
- 하나의 텍스트만 부를 경우
TextLoader
사용
# pip install pypdf
loader = DirectoryLoader('./pdf',glob="./*.pdf",loader_cls=PyPDFLoader)
documents = loader.load()
len(documents) # 13
PDF :
https://arxiv.org/abs/2004.02334
Finding the Optimal Vocabulary Size for Neural Machine Translation
We cast neural machine translation (NMT) as a classification task in an autoregressive setting and analyze the limitations of both classification and autoregression components. Classifiers are known to perform better with balanced class distributions durin
arxiv.org
(3) text를 max token에 맞춰 분절
# splitting the text into
text_splitter = RecursiveCharacterTextSplitter(chunk_size=1000, chunk_overlap=200)
texts = text_splitter.split_documents(documents)
print(texts[2].page_content)
Since the parameters of modern machine learn-
ing (ML) classification models are estimated from
training data, whatever biases exist in the training
data will affect model performance. Among those
biases, class imbalance is a topic of our interest.
Class imbalance is said to exist when one or more
1Tools, configurations, system outputs, and analyses are at
https://github.com/thammegowda/005-nmt-imbalanceclasses are not of approximately equal frequency
in data. The effect of class imbalance has been
extensively studied in several domains where clas-
sifiers are used (see Section 6.3). With neural net-
works, the imbalanced learning problem is mostly
targeted to computer vision tasks; NLP tasks are
under-explored (Johnson and Khoshgoftaar, 2019).
Word types in natural language models resemble
a Zipfian distribution, i.e. in any natural language
corpus, we observe that a type’s rank is roughly
inversely proportional to its frequency. Thus, a
few types are extremely frequent, while most of
(4) HuggingFace Instructor Embedding
- Embedding
- OpenAIEmbedding 사용시 퀄리티는 보장하지만 비용이 증가한다. HuggingFace의 HuggingFaceInstructEmbeddings을 사용해 절약 할 수 있다.
from langchain.embeddings import HuggingFaceInstructEmbeddings
instructor_embeddings = HuggingFaceInstructEmbeddings(model_name="hkunlp/instructor-xl",model_kwargs={"device":"mps"}) # !=mac "device":"cuda:0"
# load INSTRUCTOR_Transformer
# max_seq_length 512
(5) Create DB
# Embed and store the texts
# Supplying a persist_directory will store the embeddings on disk
persist_directory = "pdf_db"
## here we are using OpenAI embeddings but in future we will swap out to local embeddings
embedding = instructor_embeddings
vectordb = Chroma.from_documents(documents=texts, embedding=embedding, persist_directory=persist_directory)
(6) persiste (사용 db 유지)
vectordb.persist()
vectordb = None
vectordb = Chroma(persist_directory=persist_directory, embedding_function=embedding)
(7) Make a retriever
retriever = vectordb.as_retriever()
(8) retriever relevant documents
- 참조할 문단 수(k=3)
docs = retriever.get_relevant_documents("What is BERT Optimal Vocabulary Size?")
len(docs) # 2
retriever = vectordb.as_retriever(search_kwargs={"k":3})
(9) Define Util
- 새로 추가된 Langchain.callback - get_openai_callback을 통해 사용량을 볼 수 있다.
import textwrap
def wrap_text_preserve_newlines(text, width=110):
lines = text.split("\n")
# wrap each line individually
wrapped_lines = [textwrap.fill(line, width=width) for line in lines]
# Join the wrapped lines back together using newlines characters
wrapped_text = '\n'.join(wrapped_lines)
return wrapped_text
def process_llm_response(text):
with get_openai_callback() as cb:
llm_response = qa_chain(text)
print(wrap_text_preserve_newlines(llm_response['result']))
print('\n\nSources:')
for source in llm_response['source_documents']:
print(source.metadata['source'])
print('\n')
print(cb)
(10) 어떤 Tokenizer를 사용했는지 질문 - (한글은 답변이 어렵다고 회피)
query = "which tokenizer use in paper?"
process_llm_response(query)
BPE (Byte Pair Encoding) vocabulary.
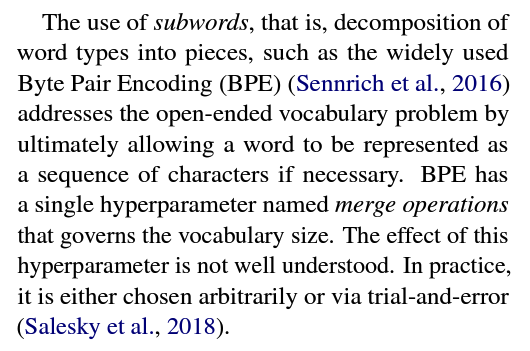
'🗣️ Natural Language Processing' 카테고리의 다른 글
[LangChain] No using OpenAI API RetrievalQA (0) | 2023.05.28 |
---|---|
[Mac] Transformer model downloaded path (0) | 2023.05.28 |
[LangChain] Building Custom Tool (0) | 2023.05.24 |
small scale text data classification (0) | 2023.05.16 |
[M1 Transformers] M1 Mac Transformers Install Error (0) | 2022.06.19 |